PERAMALAN HARGA MINYAK MENTAH MENGGUNAKAN GABUNGAN METODE ENSEMBLE EMPIRICAL MODE DECOMPOSITION (EEMD) DAN JARINGAN SYARAF TIRUAN
Abstract
ABSTRAK
Salah satu metode peramalan harga minyak mentah yang ditujukan untuk mengakomodasi sifat harga minyak mentah yang cenderung nonlinier dan nonstasioner serta dipengaruhi banyak faktor adalah peramalan yang megintegrasikan metode empirical mode decomposition (EMD) dan jaringan syaraf tiruan (JST). Dalam metode EMD, serangkaian fungsi waktu dari data masukan ditranformasikan menjadi sejumlah modus yang terdiri dari beberapa intrinsic mode functions (IMF) dan sinyal residu. Namun, metode EMD mempunyai kelemahan karena dapat menimbulkan terjadinya modus campuran dimana sebuah IMF tunggal dapat terdefinisikan menjadi beberapa sinyal dengan skala yang berbeda atau sebuah sinyal dengan skala yang sama terbentuk dalam beberapa komponen IMF yang berbeda. Dalam penelitian ini, metode EMD diganti dengan metode ensemble EMD (EEMD) dan menambahkan sinyal white noise untuk mengkompensasi modus cam-puran yang dapat terbentuk. JST berbasis feedforward neural networkdigunakan untuk memperoleh model peramalan dari masing-masing IMF dan sinyal residu. Semua IMF dan residu yang dihasilkan dijadikan masukan pada sebuah adaptive linear neural network (Adaline) untuk menghasilkan proses peramalan. Model peramalan yang telah berhasil dikembangkan dalam penelitian ini dibangun dan diuji menggunakan data bulanan harga minyak mentah West Texas Intermediate (WTI) dan Brent. Hasil uji coba menunjukkan bahwa metode peramalan yang menggabungkan EEMD dan JST menghasilkan kinerja yang lebih baik dibandingkan metode yang menggabungkan EMD dan JST. Hasil uji coba untuk data bulanan jenis minyak WTI menghasilkan RMSE sebesar 0,0330 dan Dstat sebesar 65,3061%, sedangkan untuk jenis minyakBrent menghasilkan RMSE sebesar 0,0433 dan Dstat sebesar 78,0488%.
Kata kunci: Peramalan Harga Minyak Mentah, Empirical Mode Decomposition (EMD), Ensemble EMD (EEMD), Jaringan Syaraf Tirun, Adaptive Linear Neural Network (Adaline).
ABSTRACT
One method of forecasting crude oil price which is intended to accommodate the nature of crude oil prices tend to be nonlinear and nonstationary and is influenced by many factors that integrated forecasting method empirical mode decomposition (EMD) and neural network (ANN). In the EMD method, the time series of the function of the input data being transformed into a mode that consists of several intrinsic mode functions (IMF) and the residual signal. However, EMD method has drawbacks because it can lead to a mixed mode where a single IMF can be defined into several signals with different scales or a signal of the same scale are formed in several different IMF components. In this study, EMD method is replaced with the ensemble method EMD (EEMD) and adding white noise signal to compensate for the blend mode that can be formed. ANN based feedforward neural network used to obtain forecasting model of each IMF and the residual signal. All IMF and the resulting residue used as input to an adaptive linear neural network (Adaline) to generate forecasting process. Forecasting model has been successfully developed in this study was built and tested to use monthly data price of West Texas Intermediate crude oil (WTI) and Brent. The experimental results show that the forecasting method that combines EEMD and ANN produce a better performance than methods that incorporate EMD and ANN. Test results for monthly data types WTI oil yield of 0.0330 and a RMSE of 65.3061% dstat, while Brent oil for this type produces RMSE of 0.0433 and 78.0488% of dstat.
Keyword: forecasting crude oil prices, empirical mode decomposition (EMD), ensemble EMD (EEMD), neural networks, adaptive linear neural network (Adaline)
Full Text:
PDF (Bahasa Indonesia)References
Haidar,I., Kulkarni,S., Pan, H. (2008), “Forecasting Metode for Crude Oil Prices Based on Artificial Neural Network”, IEEE.
Abosedra, S.,Baghestani, H. (2004), “On the Predictive Accuracy of Crude Oil Future Prices”, Energy Policy 32, Hal. 1389 -1393.
Yu,L.,Wang,S., Keung, K.L. (2008), “Forecasting Crude Oil Price with an EMD-Based Neural Network Ensemble Learning Paradigm”, Energy Economics 30, hal. 2623-2635.
Huang, N.E., Shen, Z., Long, S.R. (1998), “The Empirical Mode Decomposition and the Hilbert Spectrum for Nonlinear and Nonstationary Time Series Analysis”, Process of the Royal Society of London, A 454, hal. 903–995.
Wu, Z., Huang, N.E. (2004), Ensemble Empirical Mode Decomposition: a Derau Assisted Data Analysis Method. Centre for Ocean-Land-Atmosphere Studies. Technical Report, vol. 193, p. 51. http://www.iges.org/pubs/tech.html.
Santoso, Singgih (2009), Metode peramalan bisnis masa kini dengan Minitab dan SPSS, Elex Media Komputindo Jakarta.
Yu, L., Wang, S.Y., Lai,K.K. (2005), “A Novel Nonlinear Ensemble Forecasting Metode Incorporating GLAR and ANN for Foreign Exchange Rates”, Computers and Research 32(10), hal. 2523 – 2541.
Morana,C.(2001), “A Semiparametric Approach to Short-term Oil Price Forecasting”, Energy Economics 23 (3), Hal. 325 – 338.
Ye, M., Zyren, J.,Shore, J. (2002), “Forecasting Crude Oil Spot Price Using OECD Petroleum Inventory Levels”, International Advances in Economics Research 8, Hal. 324 – 334.
Kang, S.H., Kang, S.M., Yoon, S.M. (2009), “Forecasting Volatility of Crude Oil Markets”, Energy EconomicsI 31, Hal. 119 – 125.
Mohammadi, H., dan Su, L. (2010), “International Evidence on Crude Oil Price Dynamics :Applications of ARIMA-GARCH metodes”, Energy Economics 32. Hal. 1001 – 1008.
Guo, Zhenhai, et.al. (2012), ” Multi-Step Forecasting for Wind Speed Using A Modified Emd-Based Artificial Neural Network Metode”, Renewable Energy 37, hal. 241-249.
Kaastra, I., Boyd, M. (1996), “Designing a Neural Network for Forecasting Financial and Economic Time Series”, Neurocomputing 10, Hal. 215 – 236.
DOI: https://doi.org/10.21107/simantec.v4i1.1347
Refbacks
- There are currently no refbacks.
Copyright (c) 1970 Sri Herawati, Arif Djunaidy
Indexed By
.png)
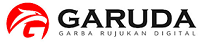
11.png)
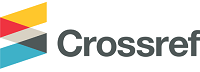
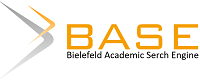
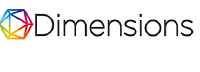
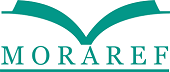
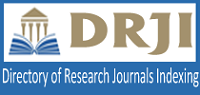