PREDIKSI PERGERAKAN HARGA SAHAM MENGGUNAKAN SUPPORT VECTOR REGRESSION
Abstract
ABSTRAK
Dalam pasar saham, harga suatu saham dapat berubah secara cepat dari waktu ke waktu. Para pemilik saham diharapkan dapat segera memutuskan kapan saham sebaiknya dijual atau tetap dipertahankan. Karenanya prediksi pergerakan harga saham sampai saat ini masih menjadi topik hangat untuk diperbincangkan dalam dunia jual beli saham. Model prediksi pergerakan harga saham yang akurat dapat membantu para investor dalam pertimbangan pengambilan keputusan transaksi saham. Di dalam praktiknya, harga suatu saham dapat diprediksi dengan menggunakankonsep analisa teknikal.Analisa teknikal didasarkan pada prinsip penggunaan data histori harga saham untuk memprediksi pergerakan saham dimasa mendatang.Tujuan penelitian ini adalah mengimplementasikan metode Support Vector Regression dalam analisa teknikal untuk memprediksi pergerakan harga saham di masa mendatang. Support Vector Regression (SVR) merupakan pengembangan dari metode support vector machine untuk kasus regresi. Metode ini mampu mengatasi overfitting serta mampu menunjukkan performa yang bagus.Dari serangkaian uji coba yang dilakukan, dapat disimpulkan bahwa metode SVR dapat memprediksi pergerakan harga saham dengan cukup baik. Hal ini terlihat dari nilai NRMSE terbaik yang didapatkan sebesar 0.14.
Kata kunci: Analisa Teknikal,Prediksi harga saham, Regresi, SVR.
ABSTRACT
Instockmarkets, the priceof a stockcanchangerapidlyover time. The investor may decide when the stock should be sold or retained.For this reason, thestockprice movementpredictionis stilla hot topicto be discussedin the world ofbuying and sellingstocks. The modelwhich was accurate forstockprice movement prediction may help investors inconsideration of the decision-making of stock transactions. In practice, stock price may be predicted with the technical analysis approach. Technical analysisis based on the principle of using historical datat o predict stock price movement in the future. The purpose of this study is to implement Support Vector Regression in technical analysis to predict the movement of stock prices. Support Vector Regression(SVR) was the development of support vector machine for regression case. This method was able to overcome the over fitting and be able to show good performance. From a series of experiments, it can be concluded that the SVR method may predict the movement of stock prices pretty well. It can be seen from the best values of NRMSE is 0,14.
Keywords: Technical Analysis, Stock price forecasting, Regression, SVR.
Full Text:
PDF (Bahasa Indonesia)References
Franses, P., & Ghijsels, H. (1999). Additive outliers, GARCH and forecasting. International Journal of Forecasting, 15(1), 1–9.
Box, G., & Jenkins, G. (1994). Time Series Analysis: Forecasting and Control (Vol. III). Englewood Cliffs: Prentice Hall.
Kim, K., & Han, I. (2000). Genetic algorithms approach to feature discretization in artificial neural networks for the prediction of stock price index. Expert Systems with Applications, 19(2), 125–132.
Armano, G., Marchesi, M., & Murru, A. (2005). A hybrid genetic-neural architecture for stock indexes forecasting. Information Sciences, 170(1):3–33.
Erkam, G., Gulgun, K., & Tugrul, U. (2011). Using artificial neural network models in stock market index prediction. Expert Systems with Applications Elsevier, 10389–10397.
Yakup, K., Melek, A., & Ömer, K. (2011). Predicting direction of stock price index movement using artificial neural networks and support vector machines: The sample of the Istanbul Stock Exchange. Expert Systems with Applications ELSEVIER, 5311–5319.
Sapankevych, N., & Sankar, R. (2009). Time series prediction using support vector machines: a survey. IEEE Computational Intelligence Magazine, 24-38.
Min, J., & Lee, Y. (2005). Bankruptcy prediction using support vector machine with optimal choice of kernel function parameters. Expert Systems with Application, 603-614.
Kim, K. (2003). Financial time series forecasting using support vector machines. Neuro computing, 307-309.
Huang, W., Nakamori, Y., & Wang, S. (2005). Forecasting stockmarket movement direction with support vector machine. Computers and Operations Research, 32(2513–2522), 2513–2522.
Murphy, J. (1999). Technical analysis of the financial markets. New York Institute of Finance.
Drucker, H., Burges, C., Smola, A., Kaufmann, L., & Vapnik, V. (1996). Support Vector Regression Machines. Advances in Neural Information Processing Systems, 155-161.
Smola, A., & Scholkopf, B. (2004). A tutorial on support vector regression∗. Statistics and Computing, 199–222.
Vapnik, V. (1995). The Nature of Statistical Learning Theory. New York: Springer-Verlag.
Karush, W. (1939). Minima of functions of several variables with inequalities as side constraints.
DOI: https://doi.org/10.21107/simantec.v4i1.1345
Refbacks
- There are currently no refbacks.
Copyright (c) 1970 Lisa Yuli Kurniawati, Handayani Tjandrasa, Isye Arieshanti
Indexed By
.png)
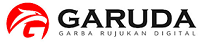
11.png)
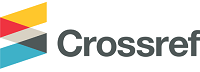
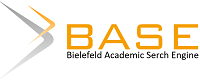
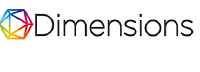
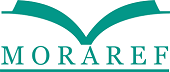
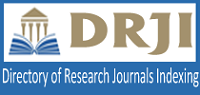