Jurnal Ilmiah Kursor is a scientific and peer-reviewed journal of Informatics Department, University of Trunojoyo Madura. It addresses issues related to the development of Computer Science or Information Technology.
ISSN: 0216-0544
Accredited S2 by Directorate General of Higher Education (SK Dirjen Dikti No. 36/E/KPT/2019) Start from Volume 10 Number 1 in 2019 until 5 years.
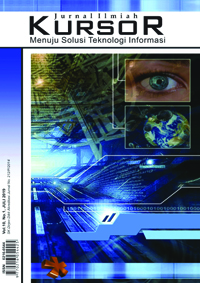
Announcements
Information |
|
Dear all authors and readers of "Kursor Journal", please kindly notice that all things related to journal submission, please access to http://kursorjournal.org/ . Thank you. |
|
Posted: 2017-10-03 | More... |
More Announcements... |